Transforming Wardrobe Management with AI-Powered Styling
Vibe is a wardrobe management app that helps users digitally organize clothing and receive AI-powered outfit recommendations.
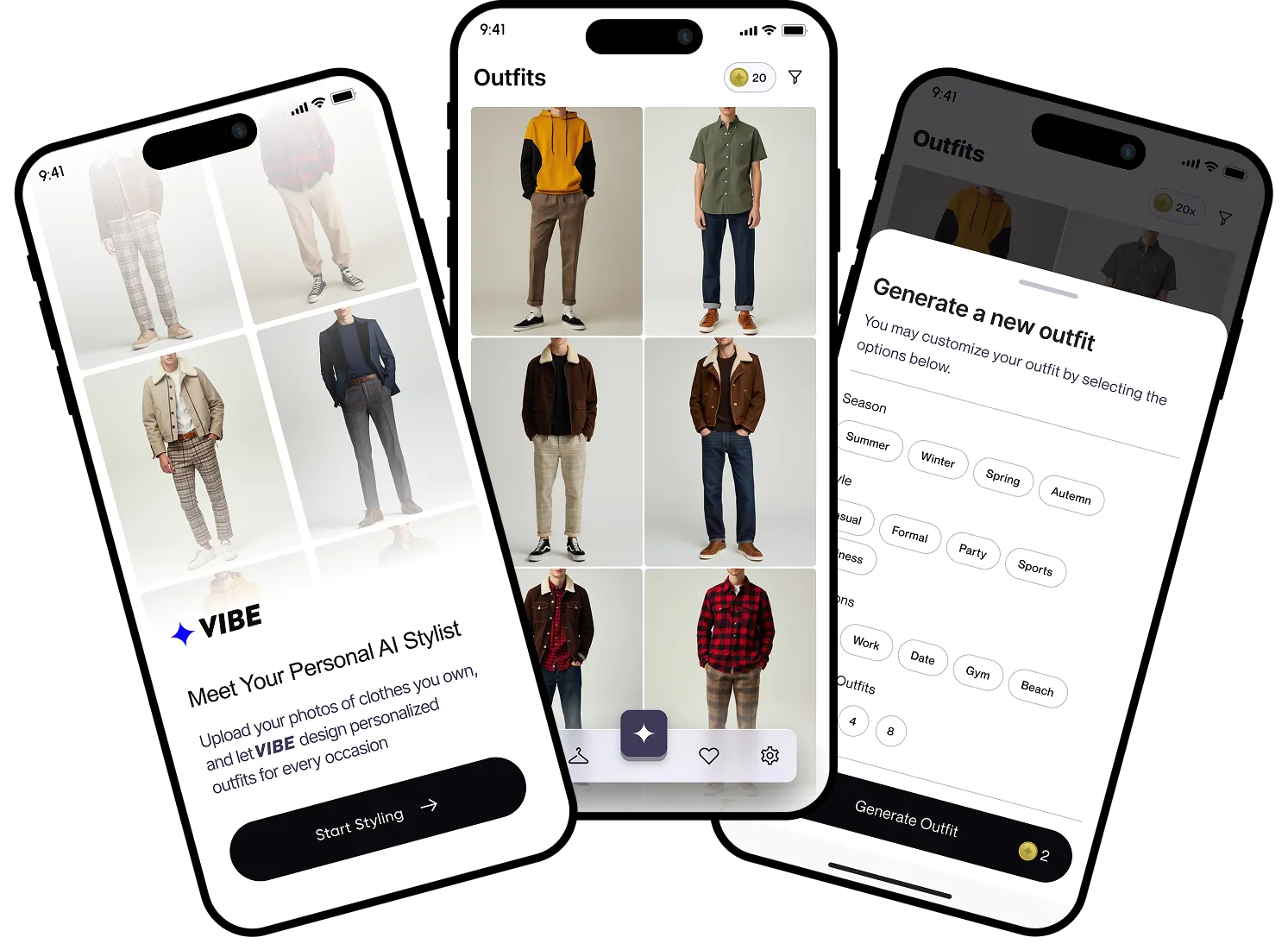
The Tech and Talent Powering Vibe
Expertise
AI & Machine Learning, Web & Mobile Development, Cloud Storage, Product & UX Design
Tech Stack
React Native, Node.js, PostgreSQL, AWS S3, OpenAI's LLM & Custom ML models, Firebase, Vercel
Deliverables
Mobile application, Web-based admin panel, Secure cloud storage, Privacy protection


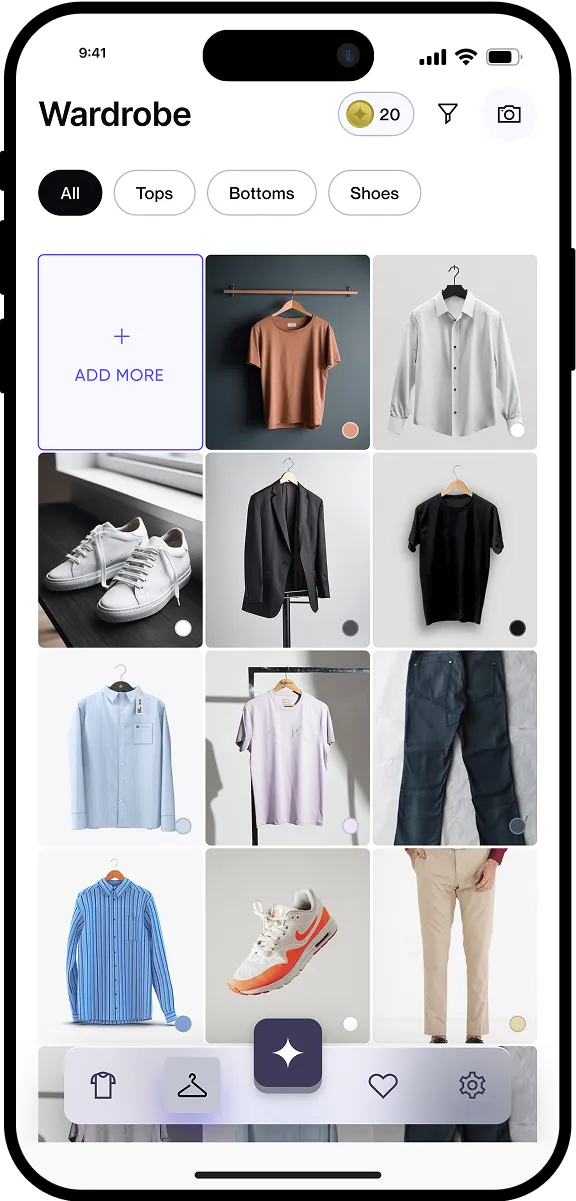



Precision Color Matching

Personalized Outfit Suggestions for Every Occasion
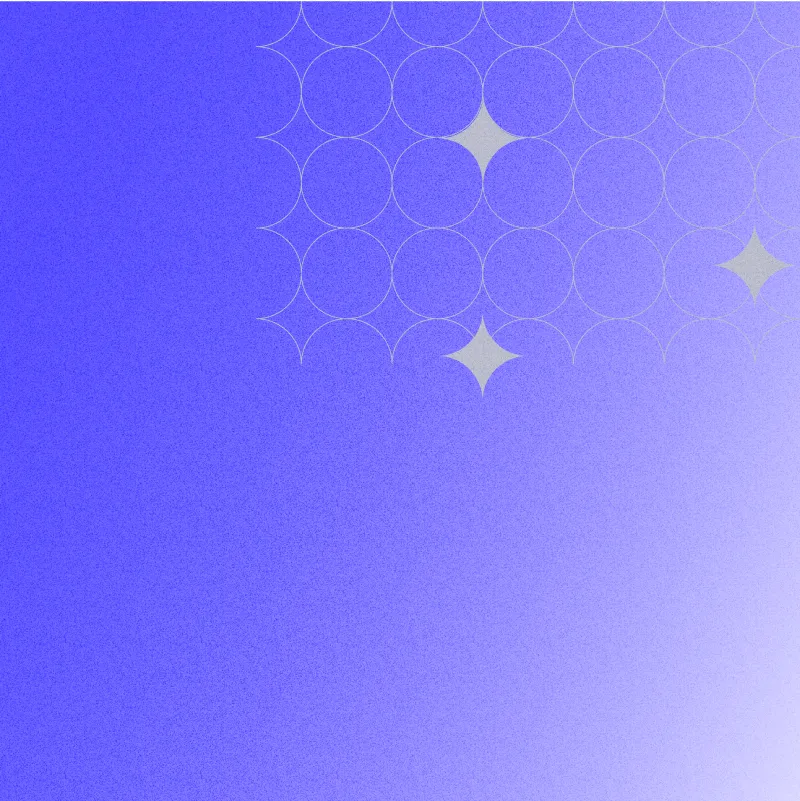


AI-Powered Wardrobe Categorization
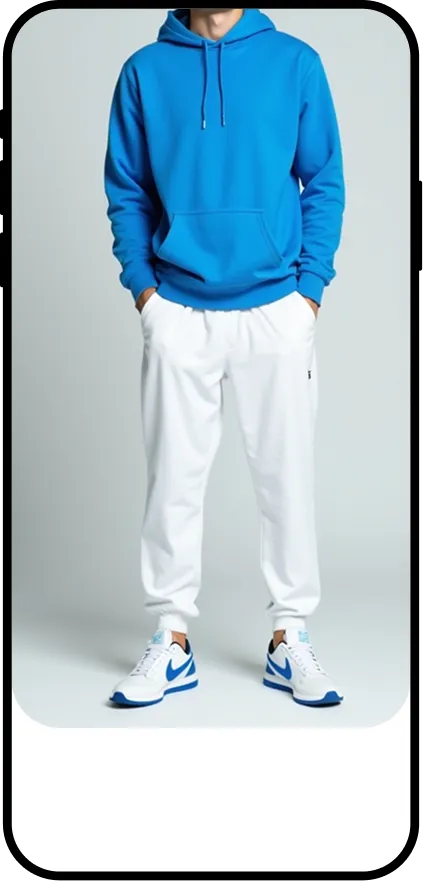

Vibe is designed to make wardrobe management effortless, blending technology with personal style to create a seamless experience. By simplifying organization and enhancing styling possibilities, it empowers users to curate their wardrobes with confidence and ease.
Seamless Wardrobe Management
Enable users to easily upload, categorize, and organize their clothing digitally.
AI-Powered Recommendations
Provide smart styling suggestions based on wardrobe contents, color compatibility, and occasions.
Privacy-First Personalization
Ensure outfit recommendations without collecting personal details like gender, age, or skin color.
Crafting an Intelligent Wardrobe Experience
Discovery & Strategy
Conducted in-depth market research to uncover gaps in wardrobe management solutions.
Design & User Experience
Developed intuitive wireframes and prototypes, ensuring a smooth wardrobe organization process.
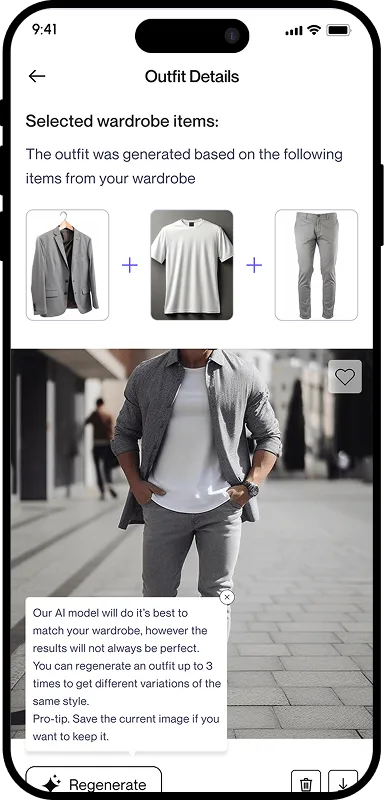

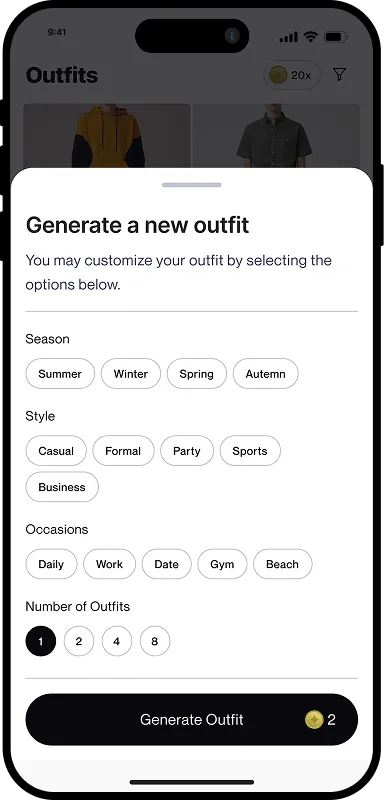
Development & Integration
Built AI-driven styling, color analysis, and cloud-based wardrobe management features.
Testing & Refinement
Optimized AI recommendations, improved color detection accuracy, and gathered user feedback for enhancements.
Refining AI for a Flawless Styling Experience


Early AI-generated outfit recommendations lacked contextual awareness, often suggesting combinations that didn’t align with fashion principles or seasonal trends. This limited the AI’s ability to provide truly relevant styling advice.
To improve this, we trained the model on color theory, fashion guidelines, and seasonal trends, enhancing its ability to curate stylish, context-aware outfit recommendations.
To solve this, we implemented OpenCV-based algorithms that analyzed highlights, mid-tones, and shadows separately, ensuring precise and true-to-life color matching.
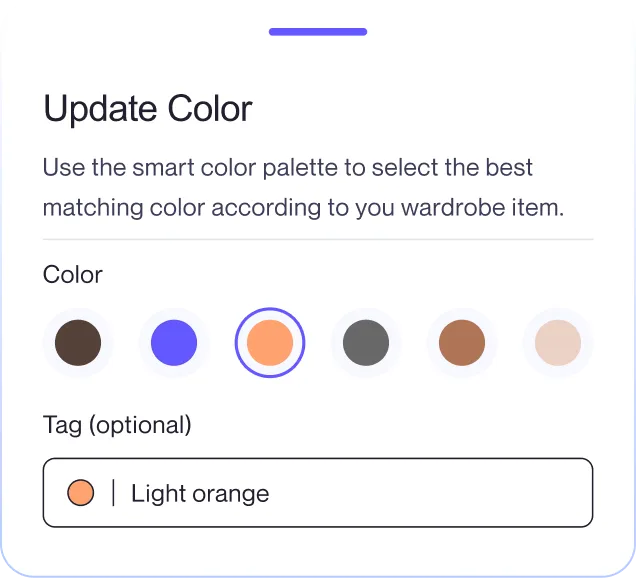